Revolutionizing Urban Mobility: The Role of Smart Sensors and AI in Optimizing eScooter Performance
The evolution of urban mobility has seen various technological advancements that cater to the growing need for efficient transportation methods. Among these, eScooters have emerged as a popular alternative, providing flexibility and convenience for short-distance travel. However, to enhance their usability and sustainability, smart escooter optimization has become critical.
The Rise of eScooters in Urban Transportation
eScooters have gained remarkable traction in urban settings, primarily due to their environmental benefit, cost-effectiveness, and ease of use. According to a report by the National Association of City Transportation Officials (NACTO), eScooter trips in the US soared to over 88 million in 2020, marking a 140% increase from the previous year. This shift in transportation method reflects broader trends towards micro-mobility.
Key Statistics on eScooter Usage
- Over 50% of eScooter users report using them instead of cars for short trips.
- Approximately 30% of eScooter trips replace walking or biking.
- The average eScooter trip length is about 1.5 miles, making them ideal for urban environments.
These statistics underscore a significant reduction in carbon emissions and traffic congestion as eScooters supplement public transit systems. As cities seek to encourage eScooter adoption, the implementation of smart technologies has emerged as a key strategy to optimize performances and user experiences.
The Role of Smart Sensors
Smart sensors integrated into eScooters facilitate real-time data collection and analysis, driving innovations in performance enhancements. These sensors monitor various parameters, including speed, battery life, user behavior, and environmental conditions. The insights gathered from this data can consequently be employed for smart escooter optimization.
Types of Smart Sensors Used in eScooters
- GPS Sensors: Track the location of the eScooter in real-time, enabling routing optimization and fleet management.
- Accelerometers: Measure acceleration forces to provide feedback on performance and safety features.
- Gyroscopic Sensors: Help in monitoring stability while riding, ensuring a safer experience for users.
- Batter Monitoring Sensors: Provide insights on remaining battery life, aiding in optimizing charging schedules and routes to prevent battery depletion.
These sensors play a critical role in the ongoing optimization of eScooter design and functionality. For instance, data collected through GPS sensors can reveal patterns in user preferences, contributing to the development of improved service areas and fleet deployment strategies.
Artificial Intelligence: Transforming Data into Insights
AI complements the functionalities of smart sensors by analyzing data to derive actionable insights. Machine learning algorithms process vast datasets collected from eScooter operations, enabling predictive analytics that inform better infrastructure and service planning.
Applications of AI in eScooter Optimization
- Predictive Maintenance: AI algorithms predict when components may fail, allowing for proactive maintenance and reducing downtime.
- User Behavior Analysis: Understanding user patterns to optimize fleet deployment and service areas.
- Dynamic Routing: AI can provide real-time routing options to optimize energy efficiency and ensure faster journey times based on traffic data.
- Battery Optimization: AI tools assess energy consumption and devise strategies for extending battery life during rides.
By leveraging machine learning, companies can refine their operational strategies and enhance rider satisfaction, making smart escooter optimization a continuous cycle of improvement driven by data.
Real-World Case Studies
Many urban areas have successfully implemented smart technologies in their eScooter programs, showcasing significant improvements in performance and user experiences. Cities such as San Francisco and Paris have emerged as leaders, adopting comprehensive plans that integrate smart sensors and AI.
Case Study: San Francisco
San Francisco’s eScooter programs utilize advanced sensor technology to gather data on usage patterns and locations, allowing the city to identify high-demand areas for deployment. Furthermore, data analytics informs operational strategies, ensuring an efficient distribution of scooters within the city.
Case Study: Paris
Paris has introduced AI-driven eScooter fleets that utilize predictive maintenance algorithms. These reduce operational costs and improve scooter availability. Additionally, the city leverages user feedback to continuously enhance service offerings, showcasing an agile response to urban mobility demands.
The Environmental Impact of Smart eScooter Optimization
The implementation of smart sensors and AI does not only cater to improving performance; it also significantly contributes to environmental sustainability. By optimizing energy consumption and encouraging scooter use over traditional vehicle reliance, eScooters become a viable solution to reduce carbon footprints in urban areas.
Benefits of eScooter Optimization on Sustainability
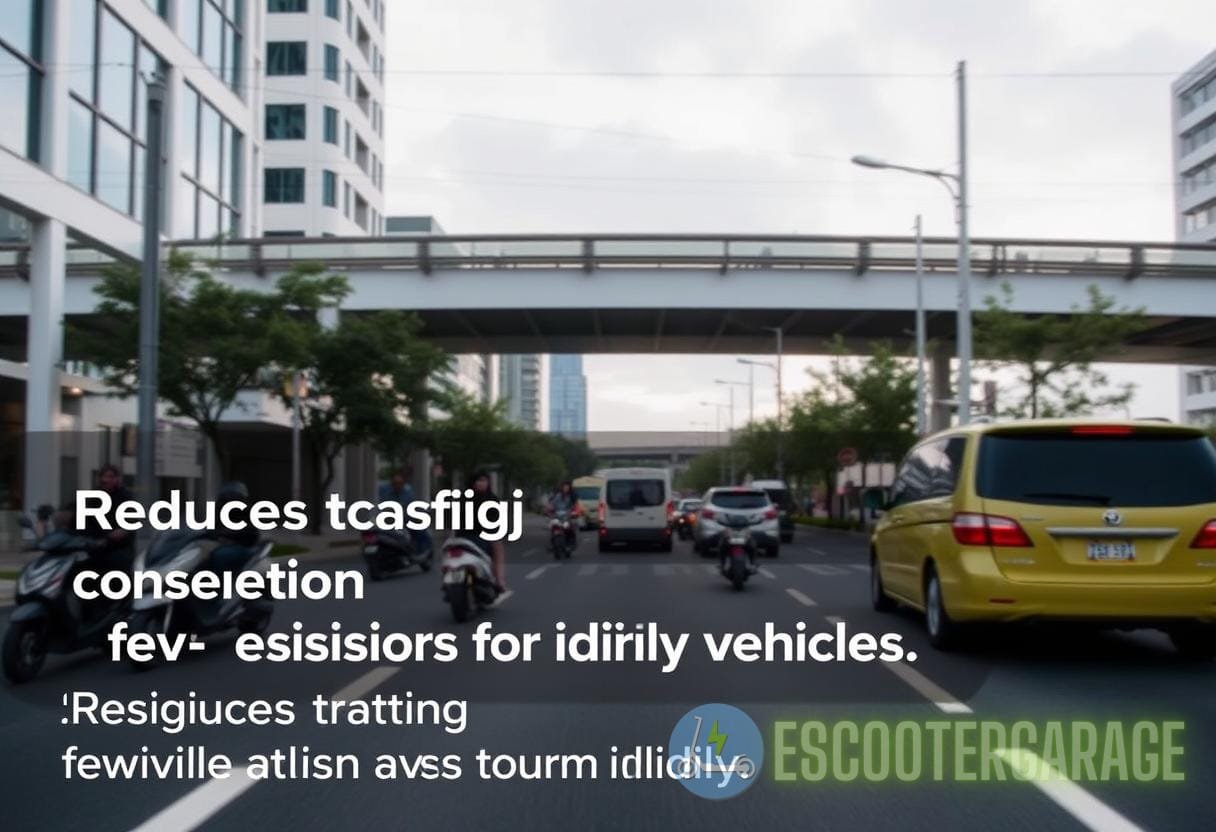
- Reduces traffic congestion, leading to fewer emissions from idling vehicles.
- Encourages the use of renewable energy sources for charging eScooter fleets.
- Aids in promoting shared mobility, reducing the number of vehicles on the road.
Smart eScooter projects contribute positively towards sustainable urban development as cities incorporate techniques to combat climate change while enhancing mobility. The emphasis on performance upgrades through smart technologies encourages more environmentally friendly transportation solutions.
Challenges in Implementing Smart Technologies
Despite the myriad benefits of integrating smart sensors and AI into eScooter systems, various challenges remain. These obstacles can hinder widespread adoption and hinder smart escooter optimization initiatives.
Key Challenges Faced
- Data Privacy Concerns: Users may be apprehensive about data tracking, necessitating transparent policies and practices.
- Infrastructure Limitations: Not all cities have the requisite infrastructure to support advanced smart sensor technologies.
- Funding and Investments: Initial setup costs for smart sensor integration and AI development can be significant.
Addressing these challenges requires collaboration between city planners, private eScooter companies, and local communities to establish effective smart mobility frameworks.
Future Trends in Smart eScooter Technology
The future of eScooter technology is interconnected with developments in smart sensors and AI. As more urban centers adopt these innovations, further advancements in smart escooter optimization will inevitably unfold.
Emerging Trends to Watch
- Integration with Public Transport: Collaborations between eScooter providers and public transport systems will enhance overall urban mobility options.
- Enhanced Safety Features: AI and smart sensors will lead to improved safety protocols, reducing accidents and enhancing user confidence.
- Blockchain Technology: Utilized for secure transactions and to enhance user safety through transparent systems.
Through these developments, smart escooter optimization is set to reshape how cities approach mobility, making it more cohesive and user-friendly.
The Role of Data Analytics in eScooter Performance
Data analytics is an indispensable aspect of optimizing eScooter performance, enabling operators and urban planners to make data-driven decisions. By analyzing patterns from user interactions, cities can better understand trends and demand, leading to focused strategies that enhance accessibility and efficiency.
Key Performance Metrics to Analyze
- Usage Trends: Analyzing peak usage times and locations to optimize deployment strategies.
- Maintenance Data: Monitoring issues to refine and improve eScooter longevity.
- User Feedback: Gathering insights from users to continually enhance service offerings.
Effective utilization of data analytics fosters enhanced smart escooter optimization, ultimately leading to superior performance and user satisfaction.
The Importance of Community Involvement in eScooter Success
Community engagement plays a critical role in the successful implementation of eScooter technologies. Involving local residents in planning and decision-making processes leads to increased acceptance of eScooter services and ultimately drives higher utilization rates.
Strategies for Engaging Communities
- Public Awareness Campaigns: Educating residents on the benefits and functionalities of eScooter services.
- Feedback Mechanisms: Involving users in sharing experiences and suggestions to foster a sense of ownership.
- Collaborations with Local Businesses: Establishing partnerships that promote eScooter use while benefitting local economies.
Engaging with communities promotes informed usage of eScooters and enhances the overall effectiveness of smart escooter optimization initiatives.
Conclusion: The Path Ahead for Smart eScooters
As urban mobility continues to evolve, smart technologies will be at the forefront of ensuring that eScooters remain a viable and sustainable transportation option. The integration of smart sensors and AI allows for comprehensive performance enhancements, driving both user satisfaction and operational efficiency. By utilizing data-driven strategies, cities can achieve substantial improvements in eScooter systems, effectively revolutionizing urban mobility for the future.